The Transparent Frontier: Balancing Creativity and Clarity in Generative AI through Explainable AI
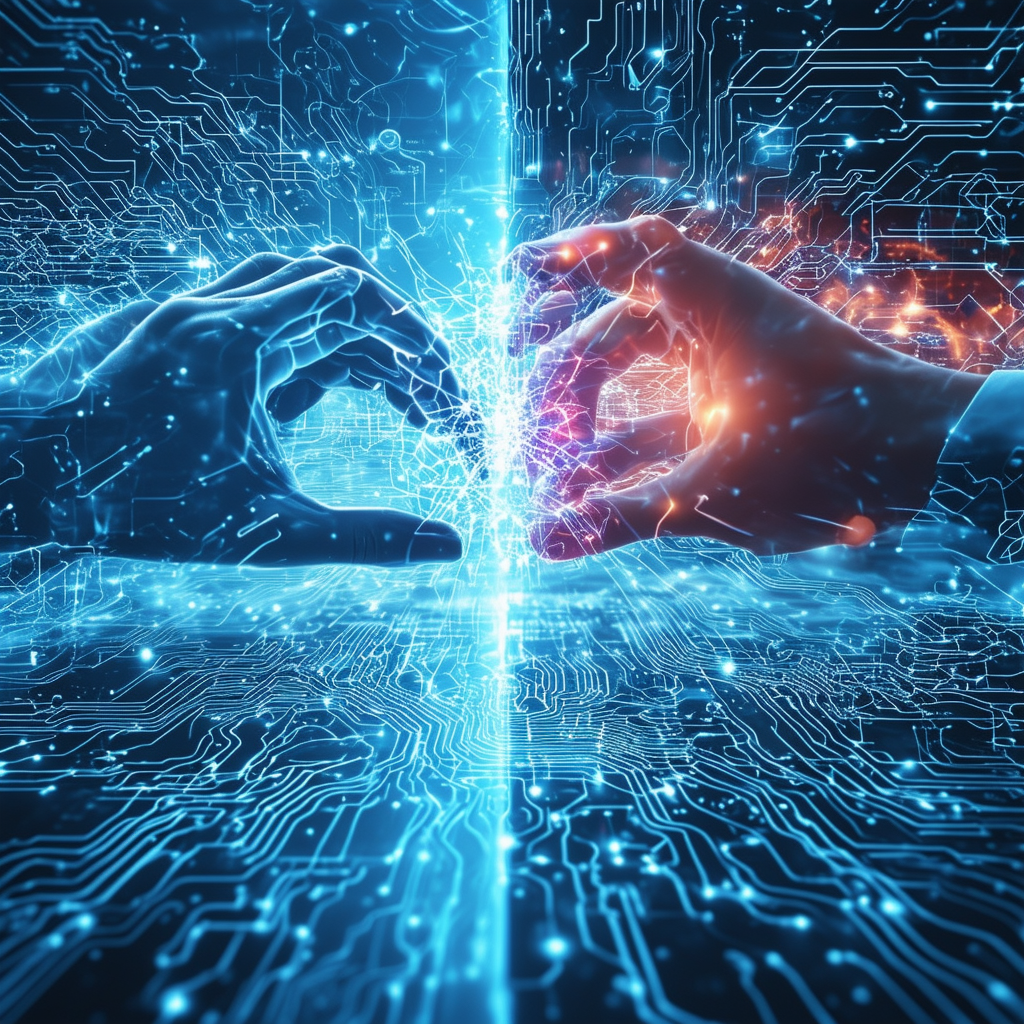
Introduction
The Current Landscape of AI Technology
Artificial Intelligence has permeated virtually every technology sector, profoundly transforming industries and daily lives. Generative AI has emerged as a particularly vibrant frontier among these transformative forces. Unlike traditional AI, which primarily analyzes data, Generative AI goes a step further—it creates. From producing realistic images and texts to generating complex music compositions and even simulating human-like conversations, the potential of Generative AI seems boundless. As organizations and creators harness this power, its applications expand, increasingly affecting verticals such as healthcare, entertainment, education, financial services, government, and more.
However, as we explore the boundaries of what machines can create, we encounter a complex web of technical, ethical, and practical challenges. How can we trust the outputs of AI that not only replicates but innovates? How can we ensure these creations are ethically aligned and free from biases that can infiltrate data sets? Results that impress their creativity and are responsibly crafted and transparent in their origins are desirable and crucial in the current AI landscape.
The Role of Explainable AI (XAI)
Introducing Explainable AI (XAI)—a subfield of AI that focuses on bridging the gap between AI capability and human understanding. In essence, XAI aims to demystify AI operations, making the processes and decisions transparent, comprehensible, and accountable. The goal of XAI is not just to inform, but to empower users, stakeholders, and regulators with the knowledge of 'how' and 'why' an AI system arrives at a particular output. This transparency is not just beneficial, but essential for user trust and adherence to international regulations and standards that demand clear explanations of algorithmic decisions.
In sectors where AI’s decisions have profound implications—like diagnosing diseases, assessing legal accountability, or executing financial transactions—the role of XAI becomes indispensable. By making these AI systems transparent, XAI facilitates trust and reliability and ensures that these technologies are used responsibly and ethically in alignment with societal values.
As we delve deeper into this article, we will explore how the synergetic integration of Generative AI with Explainable AI is beneficial and essential for harnessing the full spectrum of AI’s capabilities responsibly and ethically. Understanding this interplay and fostering its development can lead us toward a future where AI’s innovative potential is fully realized, transparent, understandable, and trustworthy.
Understanding Generative AI
Defining Generative AI
Generative AI refers to a subset of artificial intelligence technologies that have the capability to generate new content, ranging from images and text to sounds and digital environments, all based on patterns learned from vast amounts of data. Unlike traditional AI models that are primarily designed to classify or predict based on existing information, Generative AI can create novel outputs that do not directly replicate the training data. This is achieved through complex algorithms such as Generative Adversarial Networks (GANs) and Variational Autoencoders (VAEs), which enable AI to engage in an almost 'creative' process, thus expanding AI's utility from mere analysis to innovation.
Applications Across Industries
The applications of Generative AI are broad and continually evolving. In the realm of art and design, it is used to create compelling visual content, aiding artists by providing new forms of expression and tools. In media and entertainment, it powers the generation of new music tracks, video game environments, and even script writing, pushing the boundaries of creation. The healthcare sector benefits from Generative AI through its ability to model complex biological processes and generate data for new drug discovery, significantly speeding up what traditionally is a lengthy process.
Furthermore, in business applications, Generative AI is employed to generate realistic and interactive simulations for training and educational purposes, enhancing learning experiences across various fields. Another noteworthy innovation is in the technology sector itself, where AI models leverage Generative AI to produce sophisticated software codes, an advancement poised to revolutionize programming.
Challenges and Limitations
Despite its potential, the deployment of Generative AI has significant challenges. One major hurdle is quality control—ensuring the AI-generated outputs meet the requisite standards and are free from errors, a task that becomes increasingly difficult as the complexity of the outputs grows. This links closely to the ethical concerns surrounding AI-generated content, such as the potential creation of misinformation through realistic but entirely fabricated images or texts (popularly known as deepfakes). There’s also a pressing concern regarding the biases inherent in the training data, which, if unchecked, can lead to biased outputs that might perpetuate stereotypes or unfairness.
Moreover, there is an ongoing debate about intellectual property rights concerning the outputs generated by AI, complicating the legal frameworks that govern these creations. Finally, the need for transparency in how these AI models function must be balanced. As these technologies find their place in critical industries, understanding how decisions are made—particularly those that generate new content or ideas—is crucial to ensuring they are used responsibly and ethically.
As we continue to leverage Generative AI's capabilities, addressing these challenges through robust frameworks and continued innovation in related technologies, like Explainable AI, becomes imperative. This approach ensures that as we advance in our ability to create, we also progress in understanding and trusting the tools at our disposal.
The Role of Explainable AI
Defining Explainable AI
Explainable AI (XAI) is designed to make AI systems' outputs and decision-making processes transparent and understandable to humans. It encompasses a range of techniques and methodologies that elucidate how AI models operate, particularly how they arrive at their conclusions or generate specific outputs. The main goal of XAI is to foster trust and confidence in AI technologies by providing insights into their functioning, thereby demystifying the often-perceived "black box" nature of complex AI systems.
Importance in High-Stakes Sectors
The significance of XAI becomes particularly pronounced in sectors where trust and reliability are paramount. In healthcare, for instance, where AI assists in making diagnostic decisions or recommends treatments, understanding the rationale behind these suggestions is crucial for practitioners and patients. Similarly, in financial services, where AI might assess risk or manage investments, stakeholders demand transparency to ensure that decisions are sound and justifiable.
In the judicial system, where algorithmic decisions can influence outcomes that significantly affect people's lives, XAI provides the necessary transparency to scrutinize and validate these decisions, ensuring they adhere to ethical norms and legal standards. Adopting XAI in these sectors enhances operational reliability and aligns technological advancements with human values and regulatory requirements, fostering broader acceptance and integration of AI systems.
Techniques and Methods for Enhanced Transparency
XAI employs a variety of techniques to accomplish its goals:
- Model Interpretability Methods: These include simpler, more interpretable models such as decision trees and linear models that provide more straightforward explanations of their operations than more complex ones.
- Post-Hoc Explanations: Posthoc explanation techniques such as LIME (Local Interpretable Model-agnostic Explanations) and SHAP (Shapley Additive exPlanations) are used for complex models like deep neural networks. These methods offer insights after the model’s decision, explaining which features were most influential in the decision-making process.
- Visualization Techniques: Visualization tools are crucial in XAI, visually representing how AI models process inputs to produce outputs. These can include attention maps, which show parts of data (like images or text) that were pivotal in the model's decision, or t-SNE plots, which help visualize high-dimensional data in lower-dimensional spaces to better understand how data is clustered.
Each method contributes to making AI decision-making processes as clear as possible, improving the end-users understanding and fostering a more trusting relationship between human users and AI systems. As we delve deeper into the integration of Generative and Explainable AI, these techniques will be pivotal in ensuring that the innovative outputs of generative models are reliable, ethical, and understandable.
Integration of Generative AI and Explainable AI
Facilitating Understanding and Trust
The fusion of Generative AI and Explainable AI (XAI) represents a powerful synergy, particularly crucial for sectors where creative potential and operational transparency are essential. As Generative AI models become increasingly adept at producing complex and creative outputs, it becomes paramount to ensure these outputs are generated in an understandable and trustworthy manner. XAI serves this purpose by making the internal workings of Generative AI models transparent, allowing stakeholders to see what is created and how it was created.
This transparency is critical for building trust among users and stakeholders who rely on or are affected by decisions made by AI systems. For example, in areas like automated content generation, where articles, reports, and summaries are produced by AI, XAI can provide insights into the data sources and the decision-making criteria used, thus ensuring the content's accuracy and bias mitigation. This transparency is vital for maintaining the credibility and reliability of AI-generated content, particularly in news dissemination and academic publishing.
Examples
Several practical implementations of XAI in Generative AI contexts highlight its role in enhancing transparency and reliability:
- Healthcare - Drug Discovery: In the pharmaceutical industry, Generative AI models are used to create new molecular structures that could lead to effective drugs. XAI methods have been applied to these models to explain and validate the generated structures based on known chemical properties and biomedical constraints. This approach speeds up the discovery process and ensures that the models adhere to scientifically valid principles, increasing trust among researchers and regulatory bodies.
- Creative Arts - AI in Music Composition: Generative AI tools are used in music to create new compositions. XAI has enabled composers and music producers to understand the choices made by AI, such as chord progression and rhythm patterns, based on training data that includes various music genres and styles. This understanding fosters a collaborative relationship between human artists and AI, enhancing creative outcomes while retaining artistic control and transparency.
- Finance - Automated Trading Systems: Generative AI models are often employed in financial sectors to simulate different market scenarios and predict stock movements. XAI has made these simulations transparent to investors and regulatory agencies. XAI builds confidence in these automated systems by explaining how variables and historical data are used to predict trends, which is crucial for their acceptance and widespread use.
These case studies underscore the essential role of XAI in bridging the gap between the advanced capabilities of Generative AI and the need for clear, understandable, and reliable AI outputs. As industries continue to innovate and adopt AI solutions, integrating XAI will be pivotal in ensuring these technologies are used ethically, responsibly, and effectively, aligning with societal norms and regulatory standards. This integration enhances the functional capabilities of Generative AI and establishes a framework for ethical AI development, promoting a future where AI innovations are groundbreaking and grounded in transparency.
Prompt Engineering and Management
Defining Prompt Engineering and Management
Prompt Engineering and Management are emerging disciplines within the realm of Generative AI, particularly significant in the context of models like GPT (Generative Pre-trained Transformer) and other large language models. Prompt Engineering involves crafting inputs (prompts) to an AI system in a way that elicits the most accurate or desirable outputs. This practice is crucial because the quality and nature of the input significantly influence the AI's generated content.
Prompt Management extends beyond crafting inputs to include overseeing and refining these prompts based on ongoing interactions and outputs. It involves monitoring interaction patterns, adjusting inputs to enhance accuracy, and ensuring the outputs align with ethical guidelines. Together, these practices ensure that Generative AI systems perform optimally and operate within specified ethical boundaries.
Influence on Effectiveness and Ethical Implications
Prompt Engineering and Management play pivotal roles in improving the effectiveness of AI systems and overseeing their ethical implications. On the effectiveness front, well-engineered prompts can lead to more relevant and contextually appropriate outputs from AI systems. These tailored prompts allow for a more nuanced and sophisticated interaction with AI, enabling it to produce significantly more useful results for end-users. For instance, in customer service applications, a finely tuned prompt can guide an AI to provide responses that are not only correct but also aligned with the company’s tone and service ethos.
From an ethical standpoint, Prompt Management is vital. As AI systems often inherit biases present in their training data, prompt management can help mitigate these biases by adjusting inputs and guiding the AI to consider a broader, more balanced range of outputs. For example, managing prompts can help an AI avoid sensationalism or bias in news gathering and publication by steering it toward more balanced and comprehensive reporting angles.
Furthermore, in creative applications, such as AI-driven content generation, managing prompts to ensure that the AI does not generate harmful or copyrighted content is an essential ethical consideration. Companies must implement strict management protocols to ensure that their generative models produce content that respects legal and moral boundaries, such as avoiding the creation of deepfakes or other misleading information.
Overall, the strategic application of Prompt Engineering and Management in Generative AI systems incentivizes better performance and reinforces the commitment to ethical AI use. As we continue integrating AI into more facets of daily life and business, maintaining this balance of effectiveness and ethical adherence will become increasingly crucial. This necessity highlights the integral role of Explainable AI in providing the necessary transparency and insight into how AI systems, guided by human-crafted prompts, make decisions and generate outputs, ensuring they remain accountable and aligned with societal values.
Legal and Ethical Considerations
Necessity of Legal Frameworks and Ethical Standards
As Generative AI technologies advance, so must the legal frameworks and ethical standards governing their use. These frameworks are essential for ensuring that AI operations comply with the law and maintaining public trust in how these technologies are applied. Without robust legal and ethical guidelines, the deployment of Generative AI risks adverse outcomes ranging from invasions of privacy to the propagation of bias, thereby undermining the potential benefits of these technologies.
In many jurisdictions, legislation is still catching up with the fast pace of AI development. However, principles such as accountability, transparency, fairness, and respect for user privacy form the core of what these legal frameworks aim to establish. For example, the European Union’s General Data Protection Regulation (GDPR) imposes strict guidelines on data transparency and the automated processing of personal data, directly impacting how AI systems can be implemented and operated in Europe.
Importance of XAI in Compliance
Explainable AI (XAI) is a cornerstone for aligning Generative AI applications with these evolving legal and ethical standards. By making AI systems more understandable and their decisions more transparent, XAI enables compliance in several key areas:
- Transparency and Accountability: XAI techniques help to delineate how AI models function and reach specific decisions, thus supporting compliance with laws that require transparency in automated decision-making processes. This transparency is vital for accountability, allowing developers and operators to demonstrate that their AI systems act in predictable, justifiable ways.
- Fairness and Bias Mitigation: XAI can expose and help mitigate biases within AI systems, which is crucial for meeting ethical standards and legal requirements concerning fairness. For instance, XAI can reveal whether a model systematically disadvantages certain groups, which could violate anti-discrimination laws and ethical norms. Stakeholders can take corrective actions to adjust AI models and training processes by identifying these biases.
- Privacy Considerations: XAI also ensures that AI systems respect user privacy by revealing the data they use to make decisions. This aspect is critical for complying with privacy regulations that require clarity about the types of personal data collected and processed by AI applications.
As Generative AI continues to integrate deeper into societal fabrics—from healthcare and law enforcement to finance and public administration—the role of XAI becomes increasingly significant. It enhances the functionality and societal acceptance of Generative AI by making these systems more understandable and trusted. Still, it also ensures that these technologies adhere to legal and ethical standards, fostering a more responsible use of AI in our communities and industries. As we move forward, strengthening the relationship between Generative AI and XAI will be key to unlocking the full potential of AI technologies in a manner that is both innovative and aligned with fundamental human rights and values.
Future Directions and Challenges
Speculating on Future Trends
The integration of Generative AI and Explainable AI (XAI) is poised to profoundly reshape numerous domains, driving innovation while ensuring these advancements are transparent and understandable. As we look to the future, several trends are likely to emerge in this dynamic interplay:
- Enhanced Regulatory Compliance: As global regulatory bodies keep up with technological advancements, we can expect a more stringent regulatory environment around AI technologies. This will likely enhance the role of XAI in ensuring that Generative AI systems adhere to these regulations, promoting compliance, and fostering public trust in AI applications.
- Advancements in Interpretability Methods: Research in XAI will continue to evolve, leading to more sophisticated interpretability methods that can handle the increasing complexity of Generative AI models. This progress will likely include the development of standard metrics for evaluating the transparency and bias of AI systems, making these assessments more systematic and reliable.
- Broader Applications and Acceptance: As XAI improves, Generative AI will become more accessible and understandable to a broader audience, leading to its wider acceptance and application across different sectors. This could result in an explosion of creative and innovative applications of Generative AI, from more personalized healthcare treatments to more effective educational tools.
- Integration of Ethical AI Designs: Ethical considerations will increasingly be embedded into the design phase of AI development rather than being an afterthought. This proactive approach will help mitigate ethical risks early on, with XAI providing the necessary transparency for monitoring these systems.
Addressing Challenges and Research Opportunities
Despite the promising trajectory, several challenges must be addressed to realize the full potential of integrating Generative AI with XAI:
- Balancing Complexity with Clarity: As AI models become more complex, making them interpretable without compromising their performance will remain a significant challenge. Research needs to focus on developing new XAI techniques that can provide deep insights into complex AI behaviors without oversimplifying the models.
- Data Privacy and Security: With the increasing use of personal data in AI applications, ensuring data privacy and security in line with XAI will be paramount. Innovations in privacy-preserving technologies, such as federated learning and differential privacy, must be integrated with XAI frameworks to safeguard user data while maintaining transparency.
- Bias and Fairness: Despite advancements, eliminating biases from AI systems remains a formidable challenge. Continuous research is needed to develop more effective methods for detecting and mitigating biases, particularly in diverse and dynamic real-world scenarios.
- Interdisciplinary Collaboration: The future of AI will benefit greatly from increased collaboration across fields such as cognitive science, law, ethics, and data science. This interdisciplinary approach can drive the creation of more holistic AI systems that are technically sound, ethically aligned and, legally compliant.
By focusing on these areas for further research and development, we can advance towards a future where AI is powerful, innovative, responsible, ethical, and widely trusted. This balanced progress will be crucial for harnessing AI technologies' full capabilities in enhancing human lives and society.
Conclusion
Throughout this article, we have explored the dynamic interplay between Generative AI and Explainable AI (XAI), emphasizing their pivotal roles in modern technological landscapes. Generative AI continues to push the boundaries of creative and innovative capabilities, influencing numerous industries with applications that range from art generation to complex decision-making tasks. Meanwhile, Explainable AI ensures these innovations remain transparent, understandable, and trustworthy, aligning cutting-edge technology with essential ethical standards and societal values.
The critical role of XAI cannot be overstated; it acts as a bridge between the rapid advances in AI technology and the imperative to maintain ethical integrity and regulatory compliance. By making the operations of AI systems transparent, XAI helps facilitate trust and accountability, ensuring that AI technologies are used responsibly and ethically.
Integrating Generative and Explainable AI presents vast opportunities and significant challenges as we look forward. It behooves researchers, practitioners, policymakers, and stakeholders to continue their dialogue and collaboration, driving forward innovations in AI that are not only technologically advanced but are also embedded with ethical considerations. Let us engage actively in this ongoing conversation and contribute to shaping an AI-powered future that is innovative, just, and aligned with the broad spectrum of human values. Bringing these technologies to their fullest potential will require a consistent and concerted effort to ensure that the future of AI is as responsible as it is revolutionary.
Sign up for Wispera AI.
FAQ
- How do Generative AI techniques specifically handle and mitigate biases in their training data?
Generative AI typically addresses biases in training data through various strategies to identify and mitigate skewed data inputs before they negatively affect the output. One common approach involves diversifying the training datasets to include a broader spectrum of examples that more accurately represent the variability found in real-world scenarios. Another strategy involves using algorithms designed to detect and correct biases by adjusting how data is weighted and interpreted during the learning process. For example, adversarial training can help expose and mitigate biases by challenging the model to perform well against deliberately designed adversarial inputs that attempt to expose model weaknesses.
- What are some examples of failures or limitations of Explainable AI (XAI) techniques when applied to complex Generative AI models?
While Explainable AI (XAI) significantly bridges the gap between AI operations and human understanding, it poses challenges, especially with complex models such as deep neural networks. One significant limitation is that the more complex the model, the harder it is to generate clear and intuitive explanations that accurately reflect how decisions are made. XAI methods like LIME (Local Interpretable Model-agnostic Explanations) or SHAP (Shapley Additive exPlanations) can offer insights, but these are often only approximations of the model's reasoning. Moreover, these techniques can sometimes provide contradictory explanations depending on the method and parameters used, which can confuse rather than clarify. Additionally, computational efficiency is challenged as XAI can require extensive additional processing, which might not be feasible in time-sensitive applications.
- Can you elaborate on the legal implications for intellectual property rights concerning AI-generated outputs?
The legal implications concerning intellectual property rights for AI-generated outputs primarily focus on determining who holds the copyright for works created not by humans but by an AI system. Currently, the legal framework in many jurisdictions is geared towards creations by human authors, leaving a significant grey area regarding AI. Some argue that the developers or owners of the AI should hold the copyrights, whereas others suggest a new category might be needed for AI-generated content. This debate continues as technological capabilities and legal systems evolve to adapt to new realities. Countries and governing bodies have begun exploring amendments to copyright laws. Still, as of now, there isn't a universally accepted standard, causing inconsistencies in how AI-generated works are treated globally. This presents a complex scenario for creators, users, and legal experts as they navigate copyright protection, usage rights, and the monetization of AI-generated content.